The Artificial Intelligence in Medicine (AIM) project aims to exploit the expertise of INFN and associated researchers on medical data processing and enhancement, and turn it in the development of advanced and effective analysis instruments to be eventually clinically validated and translated into products.
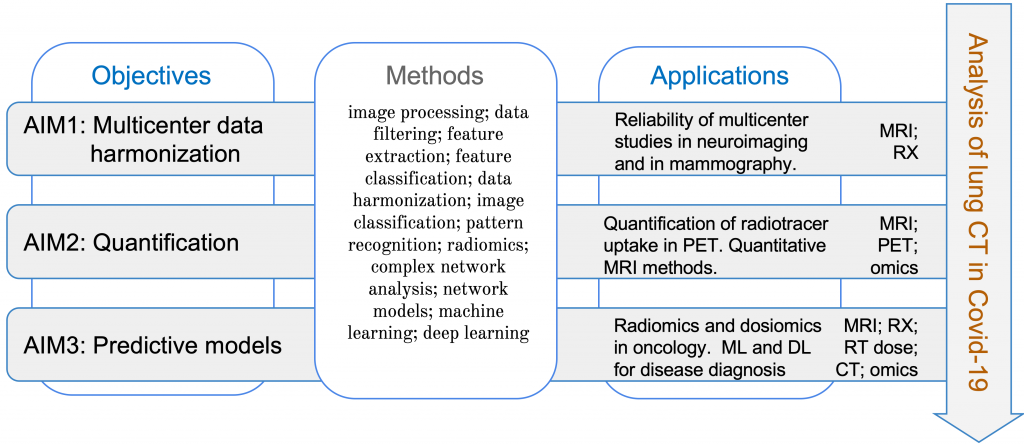
AIM1: Data harmonization
AIM1 is focused of data harmonization problems, and includes the development, optimization and implementation of algorithms (including ML- and DL-based ones) devoted to the management of the systematic errors introduced by different acquisition machine (e.g. MR scanners, RX tubes, etc.) in multicenter studies. Case studies include a large data samples of multicenter structural and functional MRI data, and a multicenter data sample of breast mammographic (screening and clinical) and Digital Breast Tomosynthesis (DBT) images. Also the integration of high-throughput data from different platforms (e.g. microarray and Next-Gen Sequencing) will be relevant for this aim.
AIM2: Quantification
AIM2 deals with methodological developments in extracting quantitative information that can directly relate-to / impact-on a clinical outcome. Quantification does not usually involve ML or other data mining tools that need validation on an external dataset. Rather, it seeks direct data transformations either onto a small set of indexes (typically within dimensionality reduction techniques) that have direct and unequivocal clinical meaning, or directly into physically measurable quantities. Effective developments rely on the incorporation of external knowledge (i.e. clinical expertise/ex vivo/phantom measurements) rather than on a data-driven approach (typical of ML). Quantification methods need to be both robust (with respect to provenance and data acquisition protocols) and applicable to small and large dataset alike.
AIM3: Predictive models
AIM3 has the objective of putting in place predictive medicine solutions, and it includes at present: the search of biomarkers of pathology in a variety of disease conditions investigated with the most informative and appropriate diagnostic modalities (e.g. RX, MRI, PET); the identification of drug targets by in-silico modelling personalized with patient profile; the identification of predictive features of treatment response in trans-cranial-MR-guided Focused Ultrasound Surgery; the prediction of treatment outcome in oncologic patients, relying on CT, MRI, and clinical dosimetric data of patients.